Here is a working example of the code, and the resultant output:
from jax import config
config.update("jax_enable_x64", True)
import celerite2.jax
import jax.numpy as jnp
import matplotlib.pyplot as plt
import numpy as np
import numpyro
import numpyro.distributions as dist
from celerite2.jax import terms as jax_terms
from jax import random
from numpyro.infer import MCMC, NUTS, Predictive
np.random.seed(42)
prior_sigma = 2.0
freq = np.linspace(1.0 / 8, 1.0 / 0.3, 500)
omega = 2 * np.pi * freq
t = np.sort(
np.append(
np.random.uniform(0, 3.8, 57),
np.random.uniform(5.5, 10, 68),
)
)
yerr = np.random.uniform(0.08, 0.22, len(t))
y = 0.2 * (t - 5) + np.sin(3 * t + 0.1 * (t - 5) ** 2) + yerr * np.random.randn(len(t))
true_t = np.linspace(0, 10, 500)
true_y = 0.2 * (true_t - 5) + np.sin(3 * true_t + 0.1 * (true_t - 5) ** 2)
def numpyro_model(t, yerr, y=None):
mean = numpyro.sample("mean", dist.Normal(0.0, prior_sigma))
log_jitter = numpyro.sample("log_jitter", dist.Normal(0.0, prior_sigma))
log_sigma1 = numpyro.sample("log_sigma1", dist.Normal(0.0, prior_sigma))
log_rho1 = numpyro.sample("log_rho1", dist.Normal(0.0, prior_sigma))
log_tau = numpyro.sample("log_tau", dist.Normal(0.0, prior_sigma))
term1 = jax_terms.SHOTerm(
sigma=jnp.exp(log_sigma1), rho=jnp.exp(log_rho1), tau=jnp.exp(log_tau)
)
log_sigma2 = numpyro.sample("log_sigma2", dist.Normal(0.0, prior_sigma))
log_rho2 = numpyro.sample("log_rho2", dist.Normal(0.0, prior_sigma))
term2 = jax_terms.SHOTerm(sigma=jnp.exp(log_sigma2), rho=jnp.exp(log_rho2), Q=0.25)
kernel = term1 + term2
gp = celerite2.jax.GaussianProcess(kernel, mean=mean)
gp.compute(t, diag=yerr**2 + jnp.exp(log_jitter), check_sorted=False)
numpyro.sample("obs", gp.numpyro_dist(), obs=y)
numpyro.deterministic("psd", kernel.get_psd(omega))
nuts_kernel = NUTS(numpyro_model, dense_mass=True)
mcmc = MCMC(
nuts_kernel,
num_warmup=1000,
num_samples=1000,
num_chains=2,
progress_bar=False,
)
rng_key = random.PRNGKey(34923)
mcmc.run(rng_key, t, yerr, y=y)
posterior_samples = mcmc.get_samples()
t_pred = jnp.linspace(0, 10, 500)
predictive = Predictive(numpyro_model, posterior_samples, return_sites=["obs"])
rng_key, rng_key_pred = random.split(rng_key)
predictions = predictive(rng_key_pred, t=t_pred, yerr=jnp.mean(yerr))
predicted_means = predictions["obs"]
mean_pred = jnp.mean(predicted_means, axis=0)
lower_ci = jnp.percentile(predicted_means, 2.5, axis=0)
upper_ci = jnp.percentile(predicted_means, 97.5, axis=0)
plt.figure(figsize=(10, 6))
plt.plot(true_t, true_y, color="green", label="True Function", linewidth=2)
plt.errorbar(t, y, yerr=yerr, fmt=".k", capsize=3, label="Observed Data")
plt.plot(t_pred, mean_pred, color="blue", label="Predicted Mean", linewidth=2)
plt.fill_between(
t_pred, lower_ci, upper_ci, color="blue", alpha=0.3, label="95% Credible Interval"
)
plt.xlabel("t")
plt.ylabel("y")
plt.title("Posterior Predictions with 95% Credible Intervals")
plt.legend()
plt.grid()
plt.show()
With emcee the predictions look like:
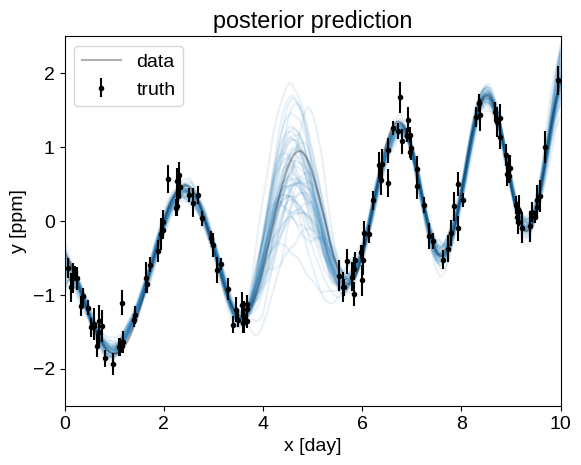
Can anyone spot what I am doing wrong, or explain how to get correct predictions from numpyro using Predictive?